Insights
From stratospheric antennas to implantable bio-sensors, from the thermodynamics of carbon capture to quantum technology and AI-designed biology, we use deep tech to challenge and change the status quo.
Read our latest research and insights to help you see beyond the innovation hype and find out how emerging technologies are solving important business problems.
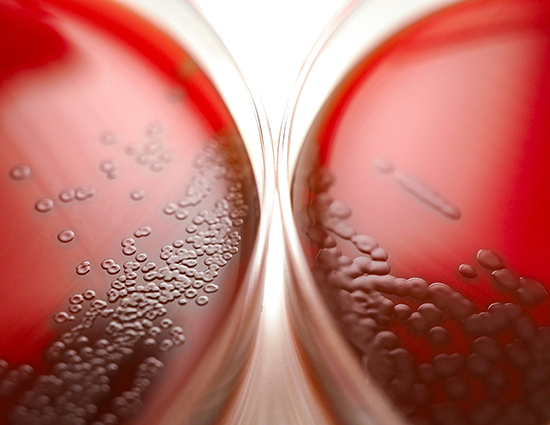
Primary care diagnostics are key to addressing antimicrobial resistance
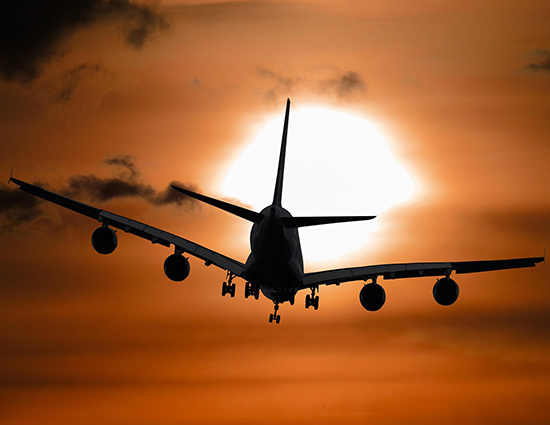
SpaceNews: Deep tech is driving the next frontier in in-flight connectivity
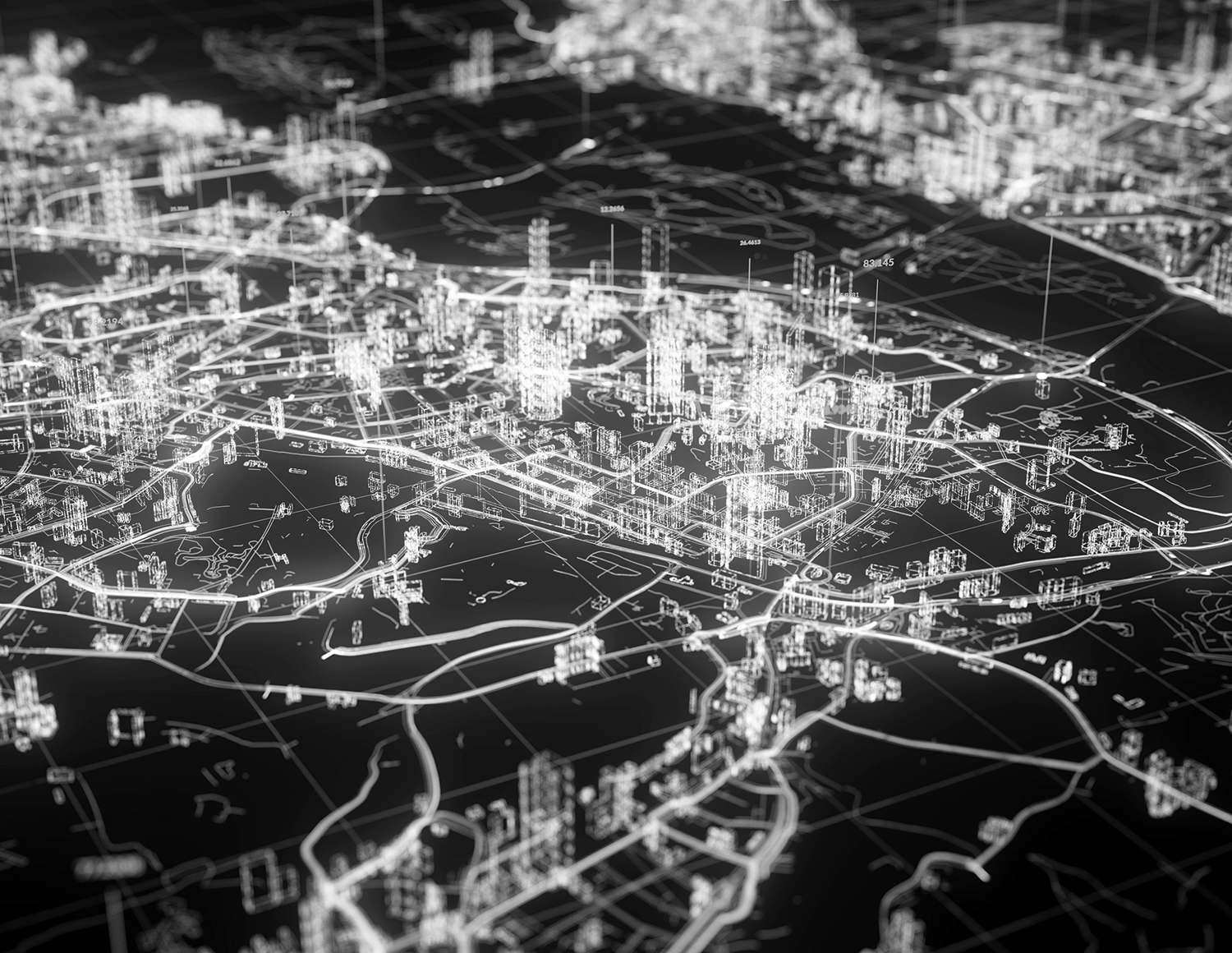
Plug in, cash in: Pathways to accessing billions of untapped value in the future grid
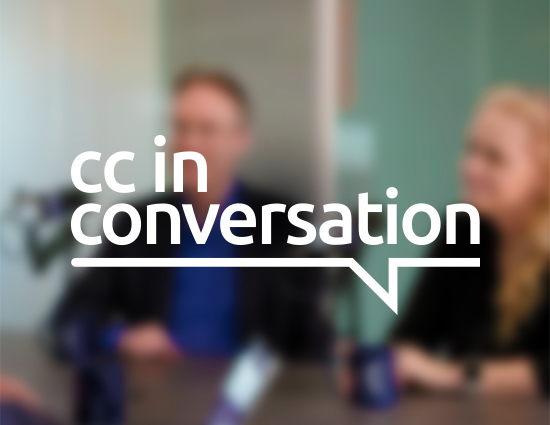
Creating business value while connecting the world – the inside view from Iridium
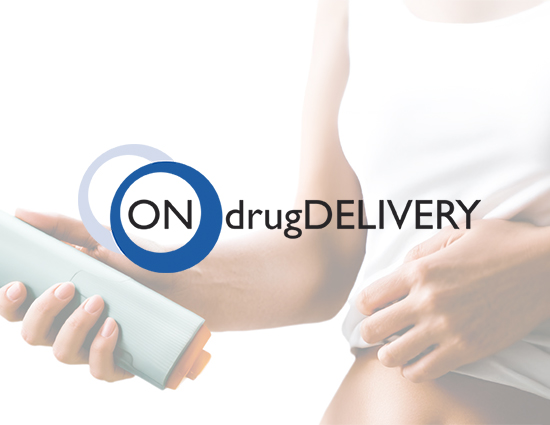
Next generation autoinjectors: balancing Patient, Pharma, Payers and Planet
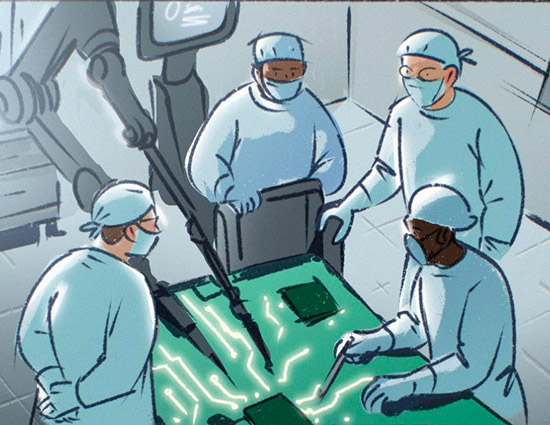
Software eats the operating room?
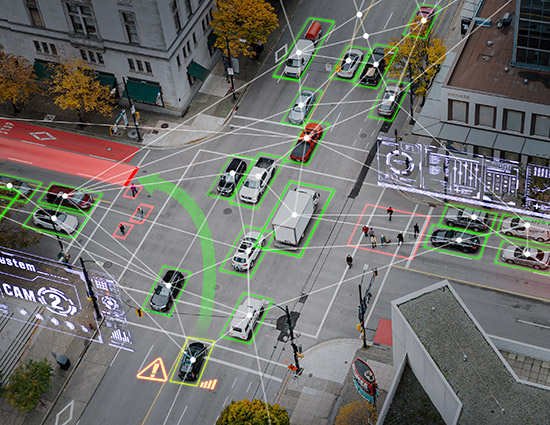
Podcast: Autonomous AI Technologies: Reshaping supply chains, workforce dynamics and society
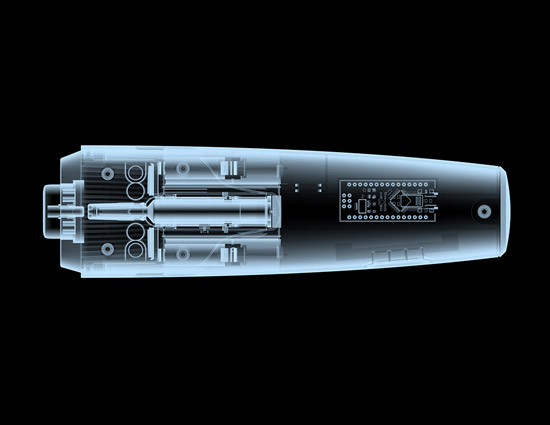
How digital twin innovation can accelerate healthcare product development
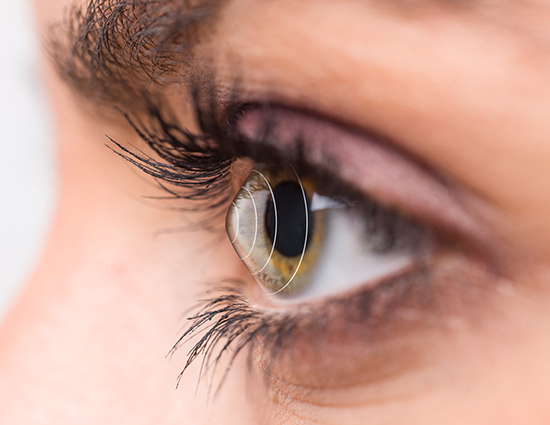