Cellular pathology screening for both research and clinical purposes presents particular challenges. The large and complicated data sets involved when looking for evidence of a tumour, say, are characterised by subtle and often elusive features – training an assistive AI system on these data requires an incredible outlay in expert supervision and access to well annotated samples which can be rare. So, it is with cautious optimism that I’m able to reveal a significant breakthrough here at CC. Our in-house research team has demonstrated the use of semi-supervised machine learning to substantially reduce the labelling requirements of such data sets. The resulting AI systems and their potential savings in time, cost and R&D risk could be game changing.
Our interest and initiatives into how AI can drive the future of digital pathology systems have been developing over some years, and this landmark on our journey is a timely one. Like the rest of the team here, I’m firmly of the view that combining the skills of clinicians with the performance of AI is the way to significantly augment decision making for pathologists working to diagnose, treat and prevent disease. Indeed, a report on ground-breaking research by Moorfields Eye Hospital, DeepMind Health and UCL alludes to the advances that are possible when clinicians and technologists collaborate.
Searching for cell morphologies
With that important theme in mind, let me break down the approach to our research initiative. As I said at the outset, pathologists searching for particular cell morphologies are faced with a huge screening load. Anything with the potential to reduce the amount of screening time by highlighting areas that are clear, questionable or highly concerning becomes hugely advantageous. And although actual clinical decision making would still be carried out by the pathologist, their workload would be massively reduced by putting AI into the loop.
The critical challenge we face is that for every type of tumour tissue, indeed any type of tissue, we look at, we need to train a new AI model. And every time we train a new model, we need an expert to establish whether it is working correctly. This could equate to thousands of hours of a pathologist’s expensive time to analyse and label the data, bearing in mind there are insufficient numbers of pathologists globally. It also needs to be carried out at an early stage of R&D, which means that organisations are incurring a large upfront cost without insight into the likely effectiveness of any given digital system. The result? High-risk, high-cost development programmes.
The fact that CC has a track record in AI and deep machine learning techniques – as well as our expertise across medical technology – meant we were well placed to search for a potential solution. Rather than an intersection of disciplines, I view our unique competency as a broad union of skills that promotes collaboration and drives progress. In this case, our focus was semi-supervised machine learning.
What is semi-supervised learning?
Most machine learning is currently supervised, involving input and target pairing labels. The system is provided with an input and an expert will provide the classification for each example. Our teams are exploring unsupervised, semi-supervised and self-supervised learning, all essentially about learning without having all data labelled. This trend is significant commercially, particularly in the feasibility phase of an AI project – in our experience on working on a large number of medical AIs, the time-consuming and expensive labelling process of input to output pairing is the primary blocker of innovation.
Just as it sounds, semi-supervised learning uses a mix of the labelled and unlabelled data. As an example, if you have 10 per cent of your data labelled and the rest is unlabelled, you’ve saved 90 per cent of your costs. The judgement to make, of course, is the level of performance against the 100 per cent expenditure.
Our approach – which we developed in collaboration with experienced pathologists – involves asking the clinician to label just a small proportion of the data. In the case of cell pathology, the data in question are digital images of staining on haematoxylin and eosin (H&E) slides, which provides the pertinent information about the pattern, shape and structure of cells in a tissue sample.
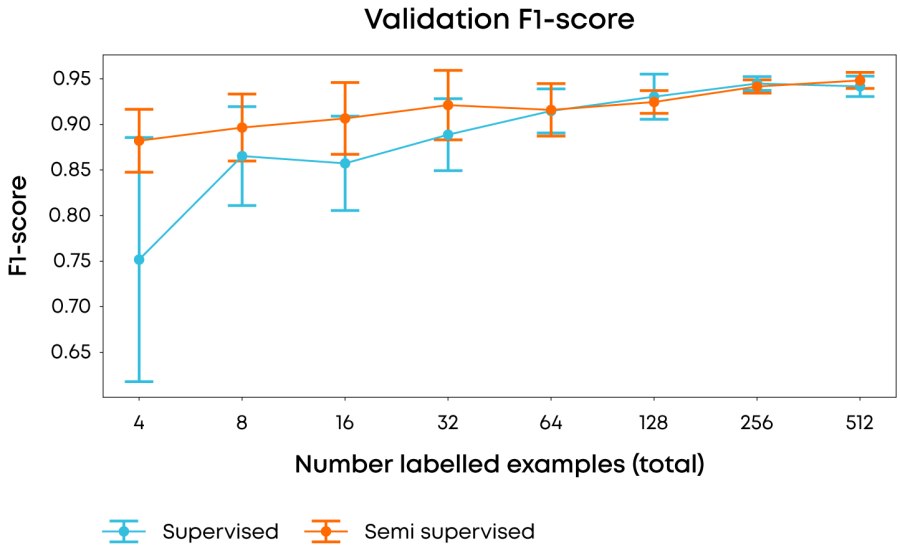
Figure 1: Comparison of average & 1σ F-1 score over 10 runs using different labelling examples in each run of the performance of fully supervised vs. semi-supervised approaches when using different numbers of labelling examples. F-1 score is used to reduce the effect on scoring of the underlying class imbalance.
During the supervised element of the process, the labelling intent of the clinician is aligned to the AI in around 10 per cent of cases. The model then determines any differences in the remaining data set, although it is not aware of their importance. But in combination with supervised labelling, the accuracy of the entire system will improve very early in the development process and there’ll be a clear indication of its likely success.
In our experiments, we used 2013 images of a single magnification factor of the Breast Cancer Histopathological Image Classification dataset1 and when using all of the data achieved state of the art performance (accuracy >85%, F1-score of 0.93) with our model, but more importantly, using our semi-supervised approach we were able to achieve an F1 score of 0.88 with only four(!) labelled samples, whilst achieving a 3-fold reduction in variance compared to a fully supervised approach. Taken together, achieving these results early in a research programme would greatly reduce the resource and time in data labelling.
Having demonstrated the effectiveness of the semi-supervised approach, we are planning to extend the project into the realm of active learning, where the learning algorithm can work with expert supervision to target the specific datapoints that add the most information to a learning algorithm, greatly increasing the rate of improvement.
Digital H&E slide images
I mentioned earlier the timeliness of the team’s achievement. One of the key factors behind our decision to pursue the project was the FDA’s acceptance in 2017 of digital slide images. It marked a big shift forward in a process that’s remained static for decades. Geographical constraints have been replaced by much wider accessibility and professionals with broad skillsets can come together to collaborate online. The physical slide no longer needs to be brought to them.
Another timely factor applies specifically to pathologists. I reiterate that this research is intended to inform systems that augment, not replace, clinicians. But the fact remains across most areas of healthcare the number of professionals is declining. Set against the societal imperative of serving a growing and aging global population with ever more complex health problems, the importance of AI-powered digital systems cannot be overstated.
The semi-supervised breakthrough complements the good deal of work we’ve carried out here at CC across a wide spectrum of deep learning and medical imaging. Previously, our Head of Intelligent Healthcare Joe Corrigan discussed our hybrid approach that uses deep learning to extract high resolution imaging from the micro motion artefacts across multiple video frames. In an earlier blog, I wrote more widely about our AI and hardware projects aimed at digitising and managing pathology specimens in a smarter, swifter way. I am pleased that are most recent progress keeps us on track to help significantly advance an area of medicine that’s changed little in the last century.
There’s plenty to discuss, so if there’s any aspect of the topic you’d like explore in more detail, do please email. I’d love to hear from you.
[1] Spanhol, F., Oliveira, L. S., Petitjean, C., Heutte, L., A Dataset for Breast Cancer Histopathological Image Classification, IEEE Transactions on Biomedical Engineering (TBME), 63(7):1455-1462, 2016. [pdf]
Expert authors
Andrew has 20 years’ experience in the Life Sciences industry, including working in the biotech industry in drug development and product development and realisation companies developing scientific instrumentation and medical devices.